In a world where technology is evolving at an unprecedented pace, the integration of Artificial Intelligence (AI) into Quality Assurance (QA) processes is not just an emerging trend—it’s an inevitable transformation. For tech enthusiasts, QA professionals, and business owners alike, understanding this evolution is crucial. The future of AI in QA promises to revolutionize how we ensure quality in software, offering efficiency, precision, and scalability like never before.
Table of Contents
The Current Landscape of AI in QA
Before we explore the future, it’s essential to understand where we are today. AI has already begun to make significant inroads into QA, enhancing traditional testing methods and processes. Tools powered by AI can automate repetitive tasks, identify patterns in vast data sets, and predict potential issues before they manifest. This shift reduces human error and accelerates the testing process, freeing QA professionals to focus on more complex, creative problem-solving tasks.
Key Applications Today:
- Automated Testing: AI-driven test automation tools can mimic human interactions with applications, conducting tests much faster and with greater coverage than manual methods.
- Predictive Analysis: By analyzing historical data, AI can predict where and when defects are most likely to occur, allowing teams to proactively address them.
- Natural Language Processing (NLP): NLP enables the parsing of user feedback and logs in natural language, transforming this unstructured data into actionable insights for QA teams.
Emerging Trends Shaping the Future
The integration of AI into QA is still in its early stages, but several emerging trends are set to define its future trajectory:
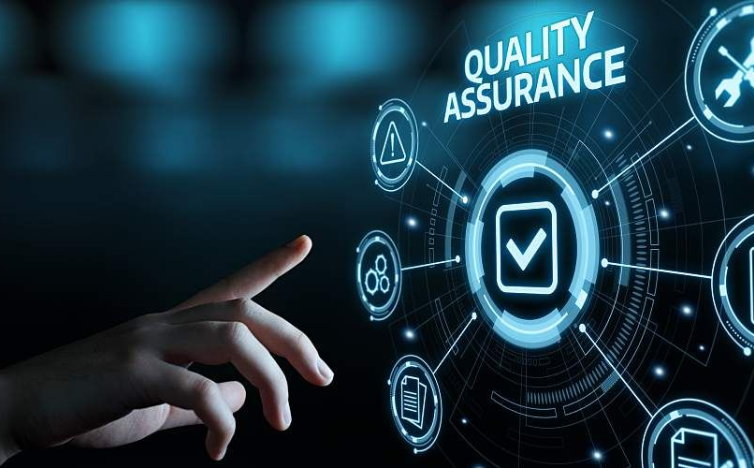
Hyper-Automation
Hyper-automation combines AI with machine learning (ML) and robotic process automation (RPA) to automate complex processes at scale. In QA, this means even more sophisticated testing scenarios can be automated, including those that involve decision-making and require adaptive responses based on real-time data.
Self-Healing Test Automation
Current automation scripts often break when there are changes in the application under test. AI introduces self-healing capabilities, where the automation framework can adapt to minor changes in the UI or code, maintaining test stability and reliability without manual intervention.
See Also: 8 Best AI Tools for the Construction Industry
Continuous Testing and Integration
AI facilitates continuous testing across the software development lifecycle. With real-time data analysis, AI can provide immediate feedback to developers, enabling rapid iteration and integration. This supports Agile and DevOps methodologies, where quick delivery cycles and constant improvement are paramount.
AI-Driven Test Generation
AI algorithms can generate test cases automatically based on user stories, requirements, or even from analyzing existing application behavior. This reduces the time and resources required to develop comprehensive test suites and ensures better coverage.
Challenges and Considerations
While the benefits of integrating AI into QA are significant, there are challenges that tech enthusiasts, QA professionals, and business owners need to consider.
- Data Quality: AI systems rely heavily on the quality and quantity of data. Poor data can lead to inaccurate predictions and unreliable test results.
- Skill Gap: QA professionals need to upskill to handle AI tools effectively, which may require substantial investment in training and development.
- Ethical and Security Concerns: Algorithms can inadvertently introduce biases, and AI systems can become targets for cybersecurity threats. It’s vital to implement robust ethical guidelines and security measures.
See Also: 10 Best AI Tools For Business
Preparing for the Future
For those ready to harness the power of AI in QA, preparation is key. Here are some steps to consider:
- Invest in Training and Development: Equip your team with the skills needed to leverage AI tools effectively. This includes understanding AI fundamentals and how they apply to QA.
- Implement AI Gradually: Start with low-risk areas to test AI solutions, allowing your team to adapt and learn before full-scale implementation.
- Focus on Data Governance: Establish strong data management practices to ensure the quality and security of the data used by AI systems.
- Collaborate with AI Vendors: Work with experienced AI solution providers who can guide your integration process and offer ongoing support.
Conclusion
The future of AI in Quality Assurance is bright and filled with potential. By automating routine tasks, enhancing predictive capabilities, and enabling continuous testing, AI is set to redefine QA processes, leading to higher-quality software and more efficient development cycles. For tech enthusiasts, QA professionals, and business owners, staying ahead of these trends and preparing for the integration of AI is not just advantageous—it’s essential for success in the digital age.
FAQs
What is the role of AI in Quality Assurance?
AI in Quality Assurance enhances testing processes by automating repetitive tasks, analyzing large data sets to predict potential issues, and enabling continuous testing throughout the development cycle. It reduces human error and allows for more focus on complex problem-solving.
How does AI improve testing efficiency?
AI-driven tools can mimic human interactions rapidly and with greater coverage than manual methods. These tools can automatically adapt to changes, self-heal test scripts, and provide immediate feedback, significantly speeding up the testing process.
What are the challenges of integrating AI in QA?
Key challenges include ensuring high-quality data for accurate AI predictions, addressing the skills gap through training in AI tools, and managing ethical and security concerns related to AI algorithms and data handling.
How can a business begin implementing AI in QA?
Businesses should start by investing in training their QA teams on AI fundamentals, implementing AI solutions in low-risk areas first to allow teams to adapt, and collaborating with experienced AI vendors for a smoother integration process.
Are there potential risks associated with AI in QA?
Some risks involve data biases introduced by algorithms, cybersecurity threats targeting AI systems, and the accuracy of predictions depending on the data quality. These risks can be mitigated with strong data governance and ethical guidelines.