In today’s digital world, data is super important for any company. It’s like the lifeblood keeping things running smoothly. Analytics tools are like special glasses that help us see clearly through all the data. These tools, powered by AI, are changing the game by giving us better insights and predictions. They make it easier for people who work with data to understand it better and make smarter decisions.
Selecting the appropriate AI-driven analytics tool can have profound implications for your business, accelerating everything from decision-making to strategic planning. In this extensive guide, we’ll walk you through the key considerations to keep in mind when choosing an AI data analytics tool, ensuring that you’re equipped with the right solution for your unique needs.
Table of Contents
Understanding the AI Data Analytics Landscape
Before choosing a data analytics tool, it’s important to understand AI’s wide range of uses. AI isn’t just one thing; it’s a bunch of different technologies with their own strengths and uses. It includes things like machine learning, which helps computers learn from data, and natural language processing, which helps computers understand human language. AI also helps with things like making predictions and cleaning up messy data. It’s like a toolbox with lots of different tools for different jobs.
Because AI tools can do many different things, you need to figure out what you want to achieve in your business first. Then, you can match those goals with the abilities of AI tools. Here are some categories to think about:
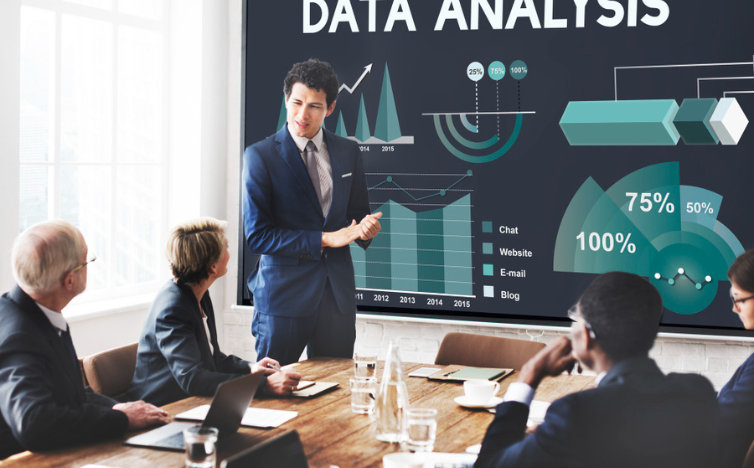
Descriptive Analytics
Understanding what has happened, usually characterized by business intelligence tools that report on past data trends.
Diagnostic Analytics
Drilling down to understand why certain events occurred by detecting patterns and relationships in the data.
Predictive Analytics
Forecasting future probability based on historical data using techniques like statistical modeling and machine learning.
Prescriptive Analytics
Advising on possible outcomes and providing recommendations on what actions to take to leverage those predictions.
Each of these categories requires different levels of AI complexity. Knowing which fits your current and future needs is pivotal to your tool selection.
Assessing Your Analytical Needs
Every business has its own unique analytical needs, and these needs typically stem from a handful of factors:
- The volume of data you wish to process
- The speed at which you need insights
- The type of analysis you require (descriptive, diagnostic, predictive, or prescriptive)
- The resources available for data management and analytics
- The level of user expertise in your organization
Start by making a list of all your data systems, any steps needed to clean or gather data, and the important insights you need for your business. This list will help you choose the right tools for what you need.
Key Features to Look For in AI Analytics Tools
Not all AI data analytics tools are created equal, and a feature that may be crucial for one organization could be excessive for another. However, there are some universal features that most businesses should look for:
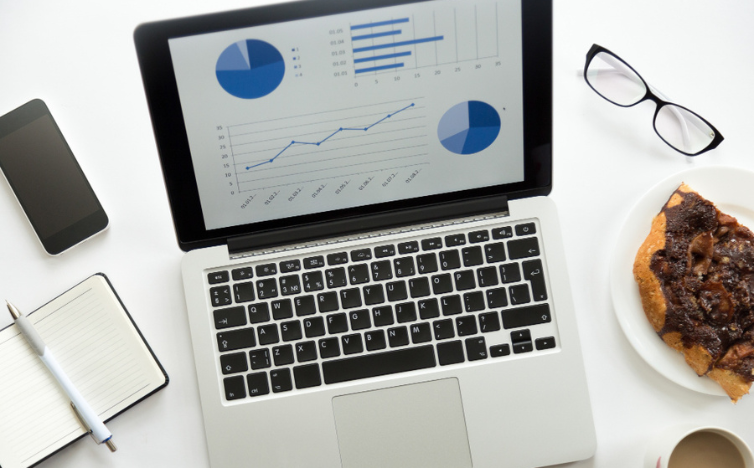
Data Integration and Preparation
How easy and comprehensive is the integration process for your existing data sources? Can the tool handle data from various sources, including structured and unstructured data?
Data Visualization
The ability to visualize data is essential for communicating insights effectively. Look for tools that offer a wide range of visualizations and are easy to use.
Scalability
Your analytics tool should be able to grow with your business’s data needs. Ensure that the tool is capable of handling larger datasets and more complex analyses as your business expands.
Ease of Use
A strong analytics tool is only helpful if it’s easy to use. Choose platforms that make complicated analyses simple with easy-to-understand interfaces and strong support.
Predictive and Prescriptive Capabilities
Depending on your need for future forecasting and recommendation engines, ensure that the tool’s predictive and prescriptive functionalities are appropriate for your industry and use case.
Deployment Options
Many tools provide cloud options, but you might require on-site or mixed deployment choices due to rules or data control needs.
Privacy and Security
With the increasing concerns around data privacy and security, make sure the tool can meet your compliance needs, including GDPR, CCPA, and any specific industry regulations.
By prioritizing these features and aligning them with your needs, you can narrow down your choices to a handful of AI analytics tools that are best-suited to your business.
Tool Selection Best Practices
Performing a side-by-side comparison of data analytics tools can be a daunting task. However, employing certain best practices can streamline the process:
Start with a Shortlist
Based on your needs and the features outlined above, create a shortlist of tools that seem to fit the bill. Consider peer reviews, analyst reports, and industry case studies to inform your shortlist.
Set Up a Proof of Concept (POC)
Once you have a shortlist, set up a POC with each tool. Test its performance with your data, evaluate usability, and gather feedback from a cross-functional team. A POC often reveals the real-world challenges and benefits that might not be apparent from marketing materials.
Consider the Total Cost of Ownership (TCO)
Analyze not just the subscription or licensing fees but also consider implementation costs, ongoing support, and the effort required for training and change management.
Look for Vendor Support
A tool’s only as good as its support. Investigate the level of customer service each vendor provides. Will they work with you to understand your business challenges, or are they simply interested in selling a product?
Common Pitfalls to Avoid
In your quest for the perfect AI analytics tool, be aware of common pitfalls that can lead to disappointments or inefficiencies:
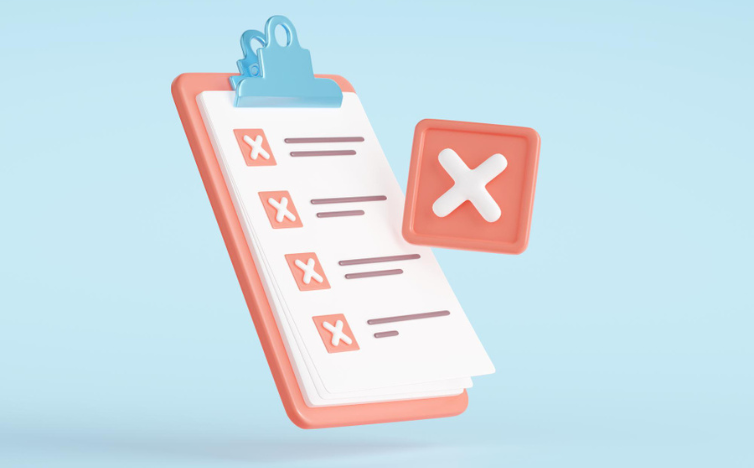
Overbuying or Underbuying
The tool that seems ‘best’ may offer a suite of features that are overkill for your organization. Conversely, underbuying may leave you with a tool that’s unable to grow with your business.
Forgetting About the End User
The opinions of the analysts and data scientists who will use the tool daily should carry significant weight in your decision-making process. After all, they will be the ones to extract value from it.
Ignoring Long-Term Business Objectives
Don’t just focus on the current moment. Consider what your business goals are for the next 5-10 years and select a tool that aligns with those long-term objectives.
Disregarding Data Governance
Ensure that the tool allows you to exercise the right level of governance over your data. With the rise in data privacy concerns, governance features are non-negotiable.
Not Preparing for Integration Challenges
Data analytics tools work best when they’re smoothly connected to other software and platforms. Start talking to the vendor early to learn about how they’ll integrate and any problems that might come up.
Conclusion
Choosing an AI data analytics tool requires a careful process. You need to understand what’s out there, figure out what you need, and thoroughly check out different options. By taking your time to look into, try out, and compare tools, you can pick one that will help your organization succeed with data. Keep in mind, the right tool isn’t just something you buy; it’s an important investment that can really boost your business.
FAQs
What is the difference between AI analytics tools and traditional data analytics tools?
AI analytics tools use complex algorithms and machine learning to process data, delivering deeper insights and predictions. Traditional tools, while effective for reporting and basic analysis, lack the advanced capabilities of AI tools.
How do I justify the investment in an AI data analytics tool to my company’s executives?
Focus on the potential return on investment (ROI) in terms of cost savings, improved operational efficiency, and better strategic decision-making. If possible, use case studies and industry benchmarks to make your case.
What kind of time frame should I be looking at for implementing an AI analytics tool?
The implementation timeline can vary significantly depending on the tool’s complexity, your data infrastructure, and the size of your organization. It’s best to get a realistic estimate from the vendor and then build in extra time for unforeseen challenges.
How can I ensure that the AI analytics tool I choose will remain relevant as technology evolves?
Choose vendors that have a history of coming up with new ideas and have a plan for making their tools even better in the future. Also, think about how flexible the tool is for keeping up with new ways of doing things and new technologies.
What role does training and upskilling play in the successful adoption of an AI analytics tool?
Training is critical. Make sure the vendor provides comprehensive training for your staff. Additionally, consider the learning curve of the tool and how you will support your team through it.