The realm of drug discovery has long been a complex, costly, and time-consuming venture. With research and development timelines often spanning over a decade and costs spiraling into billions, any innovation promising efficiency is eagerly anticipated. Enter generative AI—a technology surrounded by both buzz and skepticism. But what lies beyond the hype? Let’s unravel the tangible impacts generative AI is having on drug discovery and its potential to revolutionize the biotech and pharmaceutical landscapes.
Table of Contents
The Potential of Generative AI in Drug Discovery
Generative AI refers to a class of machine learning models designed to generate new content, ranging from text and images to, significantly, chemical compounds. In the context of drug discovery, generative AI systems can create novel molecular structures that could be developed into effective medications.
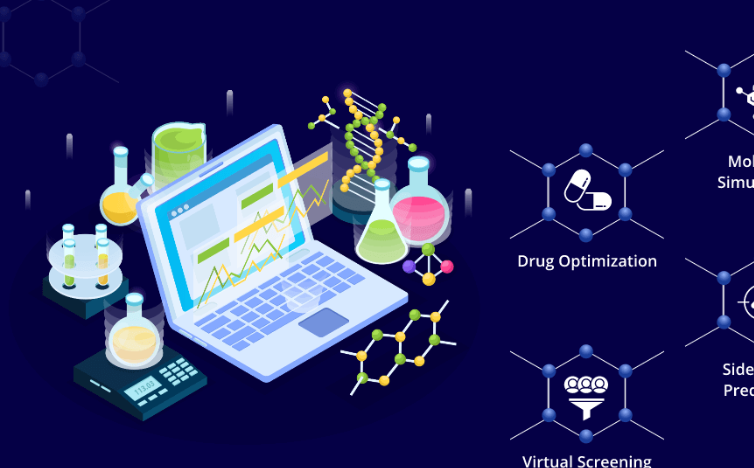
From Concept to Compound
Traditional drug discovery relies heavily on trial-and-error methods models can create molecules with specific medical benefits, so there’s less need to search through huge chemical libraries. This doesn’t just save time; it allows researchers to focus on the most promising compound candidates early in the process.
Accelerating Lead Optimization
Once a potential drug candidate is identified, optimizing its structure for efficacy and safety can take years. Generative AI tools are accelerating this phase by simulating how slight alterations to the molecule could enhance its effectiveness or reduce side effects, all in silico. This capability can significantly shorten the lead optimization stage, bringing drugs to clinical trials faster.
See Also: 8 Best AI Medical Scribes
Real-World Applications and Success Stories
Several biotech and pharmaceutical companies have already integrated generative AI into their drug discovery pipelines, yielding promising results.
Early Results
Generative AI is not just an idea; it is already showing real success. In 2020, Exscientia created a drug candidate for obsessive-compulsive disorder, which reached clinical trials in under 12 months — much faster than the usual timeline. Insilico Medicine has also gained attention for finding new compounds for fibrosis using AI, proving how useful AI can be in drug discovery.
AI is also helping in other areas of the pharmaceutical process. Companies use it to improve drug formulas and designs, predict how patients will respond to treatments, and find disease biomarkers that were hard to identify before. These early successes show that AI can help solve big challenges in drug discovery.
Challenges and Considerations
While the advancements are impressive, generative AI in drug discovery is not without its challenges.
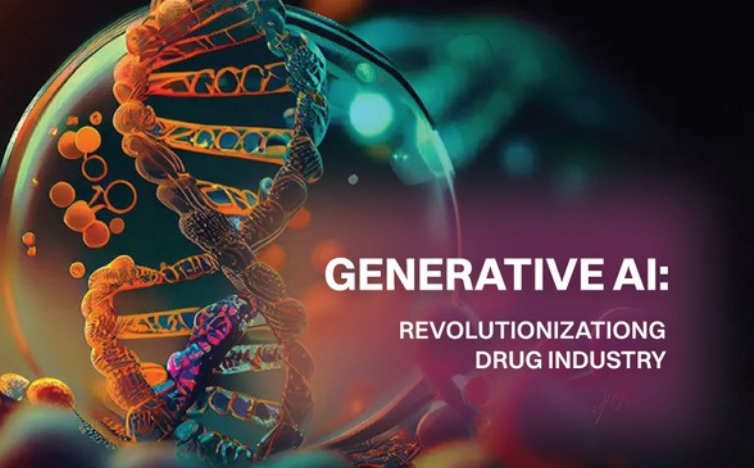
Data Quality and Accessibility
Generative AI models work best when high-quality and easily accessible data is available. Ensuring access to comprehensive, high-quality datasets remains a hurdle, as incomplete or biased data can compromise AI outputs.
Regulatory Hurdles
AI-generated drugs still need to pass through rigorous clinical trials and regulatory scrutiny. The regulatory frameworks have yet to fully adapt to AI-driven processes, posing potential delays to market access.
Ethical Implications
As with any powerful technology, ethical considerations must be addressed. The use of AI in drug discovery raises questions about privacy, consent, and the potential for misuse, necessitating transparent guidelines and oversight.
See also: 8 Best AI Tools for Transcription Services
Why It’s Difficult to Make Progress
Generative AI holds a lot of promise, but turning an AI idea into a real, effective treatment is not easy. While AI can suggest possible drug candidates, the real challenge is testing and proving these candidates through preclinical and clinical trials.
One big problem is that AI models often work like a “black box.” In traditional drug development, scientists understand each step and why a drug might work. But with AI, the results come without clear explanations, making it hard for regulators, doctors, and scientists to fully trust these outcomes.
Another issue is that the systems needed to use AI in drug discovery are still being built. AI companies and big pharmaceutical firms need to work together, but they often have different expectations. Pharma companies, which are cautious and tightly regulated, are slower to adopt AI tools, while startups expect faster progress. To unlock AI’s full potential, both sides need to agree on how to share data, follow rules, and streamline their work.
The Future Outlook
Despite the challenges, the integration of generative AI in drug discovery marks a pivotal shift in the pharmaceutical industry. By expediting the drug development process and opening new avenues for treatment, AI holds the promise of more effective and personalized medicine.
Bridging the Gap
Continued collaboration between tech developers, biotech firms, and regulatory bodies is essential to bridge the gap between AI capabilities and clinical application. Such partnerships will ensure that the full potential of generative AI is realized responsibly and effectively.
Expanding Capabilities
Future improvements in AI, especially in making it easier to understand and more reliable, will strengthen its role in drug discovery. These advancements could lead to better drug design tools that address more complex health problems.
See Also: 10 Best AI Tools For Business
Conclusion
Generative AI is indeed more than just hype in the world of drug discovery. With its ability to innovate and streamline processes, it represents a fundamental shift in how we approach and execute drug development. For biotech professionals, pharmaceutical researchers, and tech enthusiasts, understanding and harnessing the power of AI will be crucial in shaping the future of medicine.
Stay informed, stay innovative, and let’s continue to push the boundaries of what’s possible in healthcare.
FAQs
What is generative AI, and how does it apply to drug discovery?
Generative AI involves machine learning models designed to generate new data, like chemical compounds, in the case of drug discovery. This technology assists in creating novel molecules that may lead to new medications, speeding up initial phases of drug development.
How does generative AI improve the drug discovery timeline?
By designing molecules with desired characteristics and simulating their effectiveness in silico, generative AI reduces the extensive trial-and-error efforts traditional drug discovery requires, thereby accelerating both discovery and optimization phases.
Are there any successful examples of generative AI used in drug discovery?
Yes, companies like Atomwise and Insilico Medicine have seen success. For instance, Insilico Medicine discovered a treatment for fibrosis in under 46 days using AI, illustrating the technology’s capability to drastically reduce development time.
What are the major challenges faced with implementing generative AI in this field?
Challenges include ensuring data quality and accessibility, adapting regulatory frameworks to AI-driven processes, and addressing ethical concerns such as data privacy and the potential misuse of the technology.
What can we expect for the future of generative AI in pharmaceuticals?
The future likely holds increased collaboration among tech companies, biotech firms, and regulators to maximize AI’s benefits responsibly. Advancements in AI’s interpretability and robustness will expand its role in creating comprehensive drug design platforms.